Professor Rätsch, welcome to ETH
Gunnar Rätsch joined the Computer Science Department as a Full Professor of Biomedical Informatics in May 2016. Prior to accepting this position, he was conducting research at the Memorial Sloan Kettering Cancer Center in New York City, NY, USA. Professor Rätsch develops machine learning algorithms for the analysis of biological and medical data. His research will be central in ETH Zurich's initiative for personalized medicine.
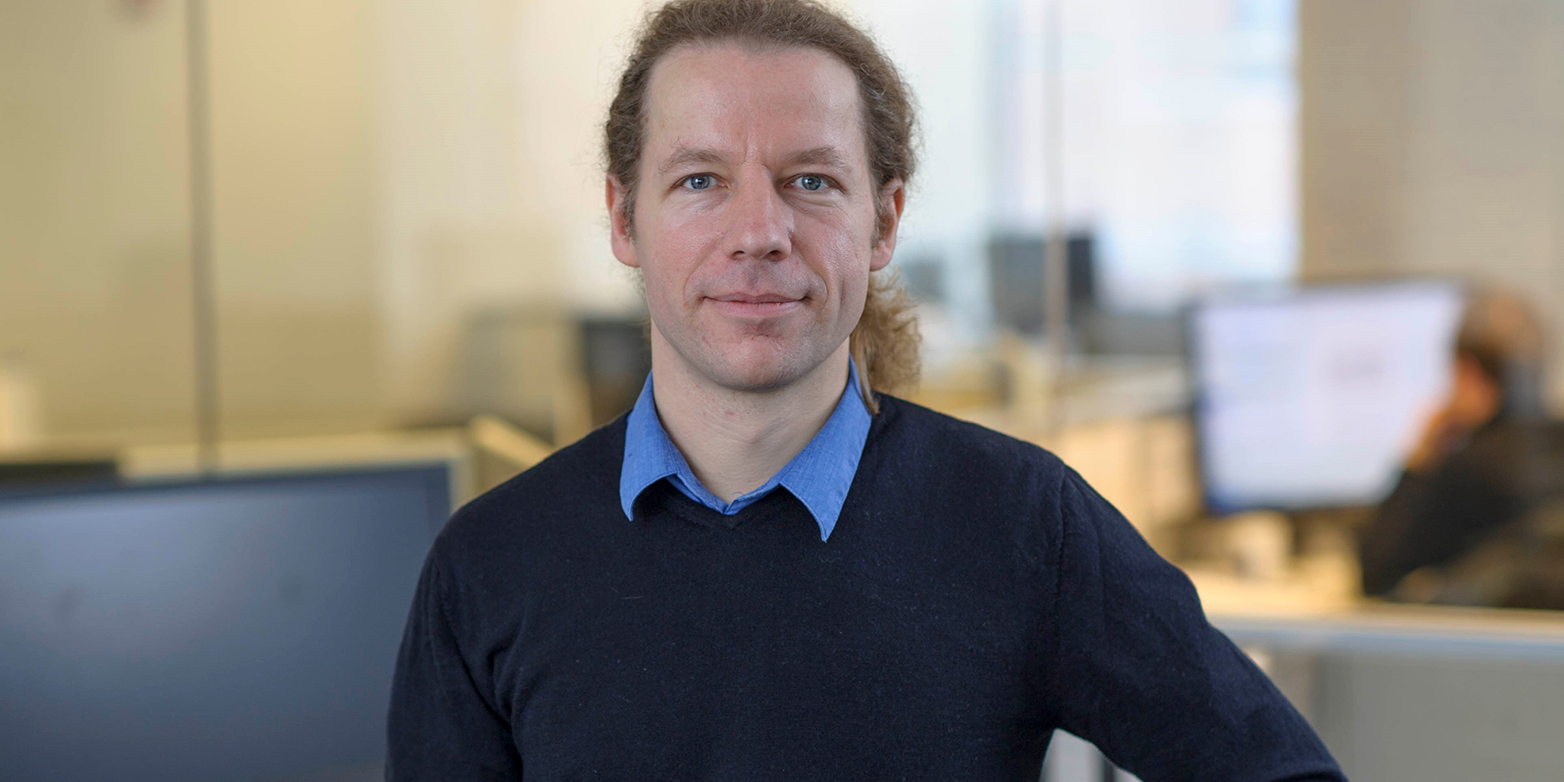
We welcome you to ETH, Prof. Rätsch. What led you to decide to begin a professorship at our university? Please describe your initial impression of ETH when you came here for your interview.
Thank you! ETH has always been an interesting place for me. About twenty years ago, when I studied computer science, I had the opportunity to spend one semester in the Department of Computer Science at ETH. It was, — and still is, — a welcoming and intellectually very stimulating place. The variety of different courses offered was exhilarating and eye-opening. Returning as a professor almost two decades later makes me feel both nostalgic and proud. The two things that have not changed since then are the ETH tradition and commitment of putting both student education and research first, which reminds me how special the school is.
Additionally, my research focuses on bridging medicine and biology with computer science. Interdisciplinary research is about forging collaborations across disciplinary and geographic borders. The proximity to the University Hospital in Zurich, the medical faculty at the University of Zurich and the planned sharing of data and tools with them, the shared infrastructure and space at the hospital, as well as the specific set-up of our projects will allow for close collaborations with short communication routes. Overall, the combination of excellent teaching and interdisciplinary research that is responsive to public needs was a key factor in my decision to join ETH.
How do you intend to bring in your background and experiences to strengthen our department?
My background is originally pure machine learning. Later I went into computational biology and medical informatics. The value my group and I can bring is a mix of technical expertise in machine learning and life sciences. All disciplines offer unique but limited perspectives into the reality they study. Our abilities to use complementary tools and resources, as well as merge different viewpoints allow us to tackle problems from diverse angles and give us the possibility to unlock new research pathways. Interdisciplinary practices are often issue- or problem-centered approaches to research that prioritize the problem at the center of research over discipline-specific concerns, theories or methods. At Memorial Sloan-Kettering Cancer Center, our previous institution, we faced the needs of being at a leading cancer research center in the US. The new research collaborations in Switzerland can lead to a set of new questions, requiring new algorithms and new hybrid research tools to be designed. As a research community, we embrace these new challenges and questions to expand the research topics and problems that we can address.
What are your current research interests?
My research interests are relatively broad because we have to cover an area from more algorithmic/ theoretical computer science to biomedical application fields. On the one hand, we work on algorithms that can learn or extract insights from data, on the other hand we develop tools that we and others employ for the analysis of large genomic or medical data sets, often in collaboration with biologists and physicians. These tools aim to solve real-world biomedical problems. In short, we advance the state-of-the-art in data science algorithms, turn them into commonly usable tools for specific applications, and then collaborate with biologists and physicians on life science problems. Along the way, we learn more and can go back to improve the algorithms.
For instance, we work on novel machine learning approaches for analyzing large collections of data from patients in intensive care units. We also work on distributed algorithms for storing, indexing and searching many petabytes large genome databases. A major challenge we are currently working on within an international collaboration is to classify certain genetic variations in the human genome, specifically in the genes BRCA1 and BRCA2, that are known to increase the risk of developing breast and ovarian cancer and make this knowledge publicly available. One barrier that we are currently facing is to get clinics and genetic testing labs on board to share their data and to then collect data that is currently stored at different institutions all over the world. These data sources together hold the potential to answer important clinical questions about the implication of a patient’s genetic variant. For patients with pathogenic variants, extra screening and other precautions may be indicated. The findings are aimed at assisting physicians in making the best treatment decision for the individual patient. In order to tackle this challenge, new informatics methods for data sharing and analysis of the internationally aggregated data are required.
How do you feel your teaching style can benefit our student population?
In most cases, we collaborate with scientists from other disciplines who work on specific biological or medical problems. This requires that the students in my lab develop a common language with scientists from other disciplines, like biology and medicine, as well as an understanding for the problems and needs that arise from these fields. Thus, the group contributes to educating a new generation of computer scientists who think and communicate across disciplinary boundaries. In the still emerging field of data science, multidisciplinarity will be a key asset for innovation. When we tackle a research question, we dig deep into computer science and try to solve the problem by implementing a technically innovative solution. We also serve as a bridge between computer science and life sciences, translating sophisticated techniques into applications. Thus, I also try to teach to think critically, independently and pragmatically — not just for the purpose of solving a complicated problem but also of solving a problem for a purpose, e.g., better treatment options for patients. This may sound ‘mainstream’ or generic but for me critical thinking — individually and collectively — is key to solve real-world problems. Often we are motivated by meaning. Contributing to something bigger than ourselves generates an even deeper sense of meaning. In my opinion smart and talented students can rise as high as their ambitions and the skills that they acquire along the way will take them there. That benefits everyone.
What do you look for in a doctoral student who wants to work under your supervision?
A doctoral student needs to be excited about research, about his/her project — otherwise it is hard to excel. In my lab, a doctoral student usually develops one or two algorithms for a problem that we have recognized as complex and relevant. Ideally this algorithm is translated into a tool that can be used to analyze biomedical data. His/her focus moves from a more abstract, theoretical, algorithmic aspect of computing to a more applied, practical and hands-on perspective. Therefore, a Ph.D. student needs to be eager to explore new technologies, as well as learn new concepts and skills to approach new multi-layered problems.
As we typically work in interdisciplinary teams, mutual understanding of researchers and practitioners with respect to each other’s needs, capabilities and goals is essential. I value team players who consult with others and share information, who are both open to unsolicited points of view, challenge established concepts, and creatively develop new approaches to problems. We have a very friendly group culture. Thus, when we hire we look at both, the technical and the interpersonal skills of a potential new team member.