Welcome, Professor Fanny Yang
28.01.2020 | Anna Ettlin
Fanny Yang officially joined the Department of Computer Science at ETH Zurich in January 2020 as Tenure Track Assistant Professor of Computer Science. Get to know her in this short interview.
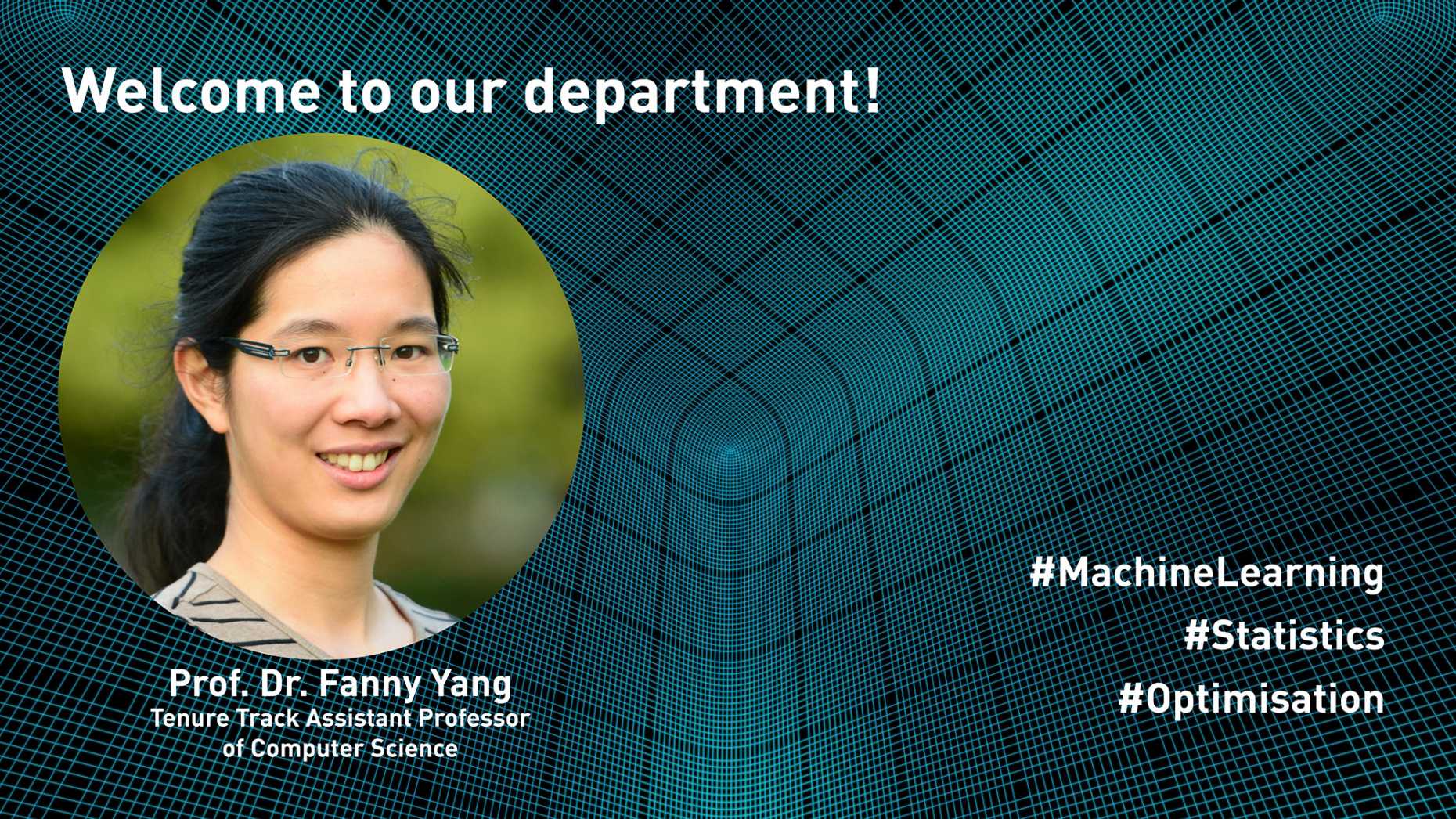
Professor Yang, welcome to ETH! What are your current research interests?
Generally speaking, I am interested in understanding and developing accurate and reliable machine learning models. To that end, I have two interconnected research agendas. First, I’d like to better understand the theoretical performance of modern machine learning algorithms and how their (robust) generalisation performance can be improved. For example, what are the fundamental conditions on the data and model space under which interpolation yields a consistent estimator, and when is regularisation useful and necessary? Classical learning and optimisation theory is insufficient to explain a variety of empirical observations arising from deep learning, and although some progress has been made on that front in recent years, we are still far from having the full picture.
Motivated by real-world applications, I’m keen to study one particular aspect that goes beyond traditional estimation and prediction performance measures: how do biases in datasets influence learning algorithms? For example, do learning procedures use inherently discriminating properties of animal species or symptoms of diseases to distinguish one from another or do they exploit spurious correlations in the training data that may not be present in test data? How can we prevent or detect the latter? Can we add information to the model output that sheds light on how the model makes predictions and how much it can be trusted? I am excited to explore all of these questions and more here at ETH!
What is the impact of your research on society?
As described above, I’m passionate about providing (provably) reliable and practical machine learning models for data scientists to use in the wild. Making these models reliable requires some measure of certainty of, and explanation for, the model prediction. For example, when an algorithm cannot classify a new medical image because it hasn’t seen the type of patient and imaging device during training, it should at least issue a warning that indicates its uncertainty. This is also important for settings where security matters. For example, when a self-driving car encounters a scenario it hasn’t seen before and is unsure about making the right decision, it should ask for human intervention. At the same time, proposed algorithms are supposed to perform well on samples that are similar to training data. My research tackles these questions from a theoretical and a practical perspective, and can therefore be potentially useful for a variety of applications in different fields.
Where were you working before you came to ETH?
I was a postdoc in the Statistics and Computer Science Departments at Stanford University and the ETH Institute for Theoretical Studies before joining D-INFK. Prior to that, I received my PhD from UC Berkeley.
Which courses will you be teaching at ETH?
My first course will be on theoretical guarantees for machine learning tailored to students who are interested in proving statistical and computational convergence rates of learning algorithms. My hope is that after this course, students can readily engage in theoretical machine learning research.
What are your first impressions of Switzerland and ETH?
Zurich is a wonderful city for a mountain and music lover like myself, and I’m really excited about exploring more of Switzerland over the next few years. I also feel very welcomed by the Swiss, even though I have yet to conquer Swiss German. My colleagues at ETH have been very supportive and helpful during my settling-in period, and I look forward to being part of this amazing group of researchers and scientists.
What advice would you give to students who are just starting out in computer science?
Computer science is a massive field, with many exciting and active areas. If students are interested in machine learning and data science, I would encourage them to become well versed in the theoretical underpinnings of machine learning as well as application areas they are passionate about. A solid theoretical foundation is crucial for understanding why and when learning algorithms work well, while familiarity with practical challenges is necessary to identify and solve problems that have a huge societal impact.