ETH Zurich scientists among the winning team at world's leading AI conference
11.06.2019
Researchers from the Max Planck ETH Center for Learning Systems and Google Research Zurich received the Best Paper Award at the International Conference on Machine Learning (ICML).
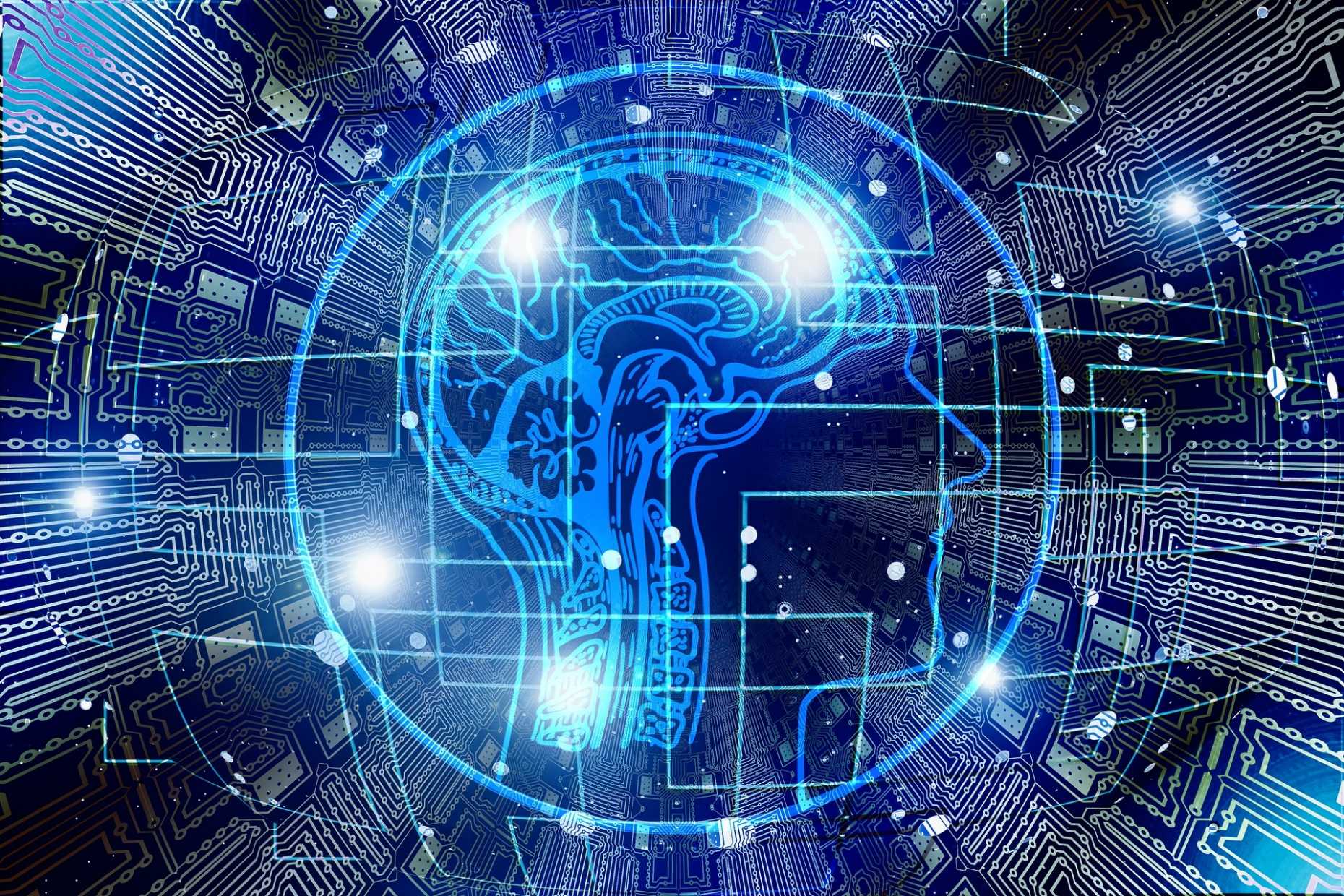
The Organizing Committee of the International Conference on Machine Learning (ICML) announced today that the Best Paper Award 2019 has gone to the authors of the publication "Challenging Common Assumptions in the Unsupervised Learning of Disentangled Representations". The research project is the result of a collaboration between scientists from ETH Zurich, the Max Planck Institute for Intelligent Systems, and Google Research Zurich. A total of 750 contributions were accepted at this year's ICML – one of the world's leading conferences in the field of machine learning. Best Paper Awards are a high distinction: they are only awarded to those whose research work will have the greatest impact in the respective field.
Francesco Locatello, a doctoral student at both Max Planck Institute for Intelligent Systems (MPI-IS) and ETH Zurich, Professor Gunnar Rätsch from ETH Zurich, Dr. Stefan Bauer, research group leader at MPI-IS, Professor Bernhard Schölkopf as well as Sylvain Gelly, Mario Lucic and Olivier Bachem, all three researchers at Google Research in Zurich, worked on the project.
"The collaboration with Google and the opportunity to use the infrastructure to train over 10.000 models has been a key advantage ". Francesco Locatello, lead author
“It has been an amazing opportunity and we hope that the follow-up work will have similar success,” said lead author Locatello. "The collaboration with Google and the opportunity to use the infrastructure to train over 10.000 models has been a key advantage ", says Locatello. "On a good desktop, this would have taken 2.5 years of continuous computation.”
Disentangled Representations
In their research, the scientists are training a computer with many thousands of images. From this large data set, deep learning approaches aim to identify patterns. An example: the researchers feed the computer thousands of images showing colored objects of different shapes. There is green, red and blue for objects like squares, hearts, circular and rectangular shapes. The images used are very simple: they have only a few pixels and are two-dimensional.
Imagine a setup where a robot arm is being used in an experimental setup. In one task, it should pick up red squares from a table. With enough trials and data, a deep neural network can be trained for the task. However, the machine fails if the researcher suddenly puts a yellow triangle in it and asks it to lift that out. The machine cannot interpret a new pattern as such. It cannot transfer what it has learned before. "That is one of the key problems in AI", says Stefan Bauer, Research Group Leader at the Max Planck Institute for Intelligent Systems. "We don't want to have to train a new Deep Net for every new color and every new shape each and every time."
A long way to go
What is child's play for a person is difficult for a machine. It is hard for it to generalize between the building blocks, to understand that one is a triangle and the other a circle – solely based on the pictures it was previously shown. "It is difficult for the machine that scans millions of parameters and recognizes patterns from them to disentangle the properties of the objects in a lower dimensional embedding (what researchers call representations). When I now present the machine with a new shape and a new color, the system is confused. The ideal would be for the system to come to the conclusion itself, without me having to train it again." But Bauer and Locatello have to disappoint those high expectations. "The dream that a machine only learns from pictures, unsupervised, without any further information (such as information that there is a red square of certain size in the image ) – that is shown to be impossible". Again, a scientist is needed to give each picture additional information. "We are far from the machine drawing conclusions on its own. The field of machine learning is very much at the beginning of a long stretch that lays ahead before we reach this goal, and additional modeling assumptions are required."
About ICML
The International Conference on Machine Learning (ICML) is the premier gathering of professionals dedicated to the advancement of the branch of artificial intelligence known as machine learning. ICML is globally renowned for presenting and publishing cutting-edge research on all aspects of machine learning used in closely related areas like artificial intelligence, statistics and data science, as well as important application areas such as machine vision, computational biology, speech recognition, and robotics.
Max Planck ETH Center for Learning Systems
In 2015, ETH Zurich and the Max Planck Institute for Intelligent Systems have joined forces in order to master the scientific challenges of learning systems by forming a unique Max Planck ETH Center. It is the first Center between the German Max Planck Society and the leading Swiss university ETH Zurich and brings together leading researchers in the field of learning systems. The goals of the Max Planck ETH Center for Learning Systems are to achieve a fundamental understanding of perception, learning and adaption in complex systems, by providing a platform for exchange in research and education.
Another goal of the Max Planck ETH Center for Learning Systems is to advance the training of young academics. Doctoral students at both institutes may profit from joint supervision through Professors of ETH and Directors of the MPI. Students and young scientists also benefit from summer schools, workshops, and internships. Offering these unique opportunities, the Max Planck ETH Center for Learning Systems ensures that young academics in the field of learning systems at ETH Zurich or at the Max Planck Institute for Intelligent Systems will have the chance of the most outstanding collaborations and exchange early on in their careers.