Welcome, Professor Theodoros Rekatsinas
Theodoros Rekatsinas joined the Department of Computer Science at ETH Zurich in May 2022 as Tenure Track Assistant Professor of Computer Science. Get to know him in this short interview.
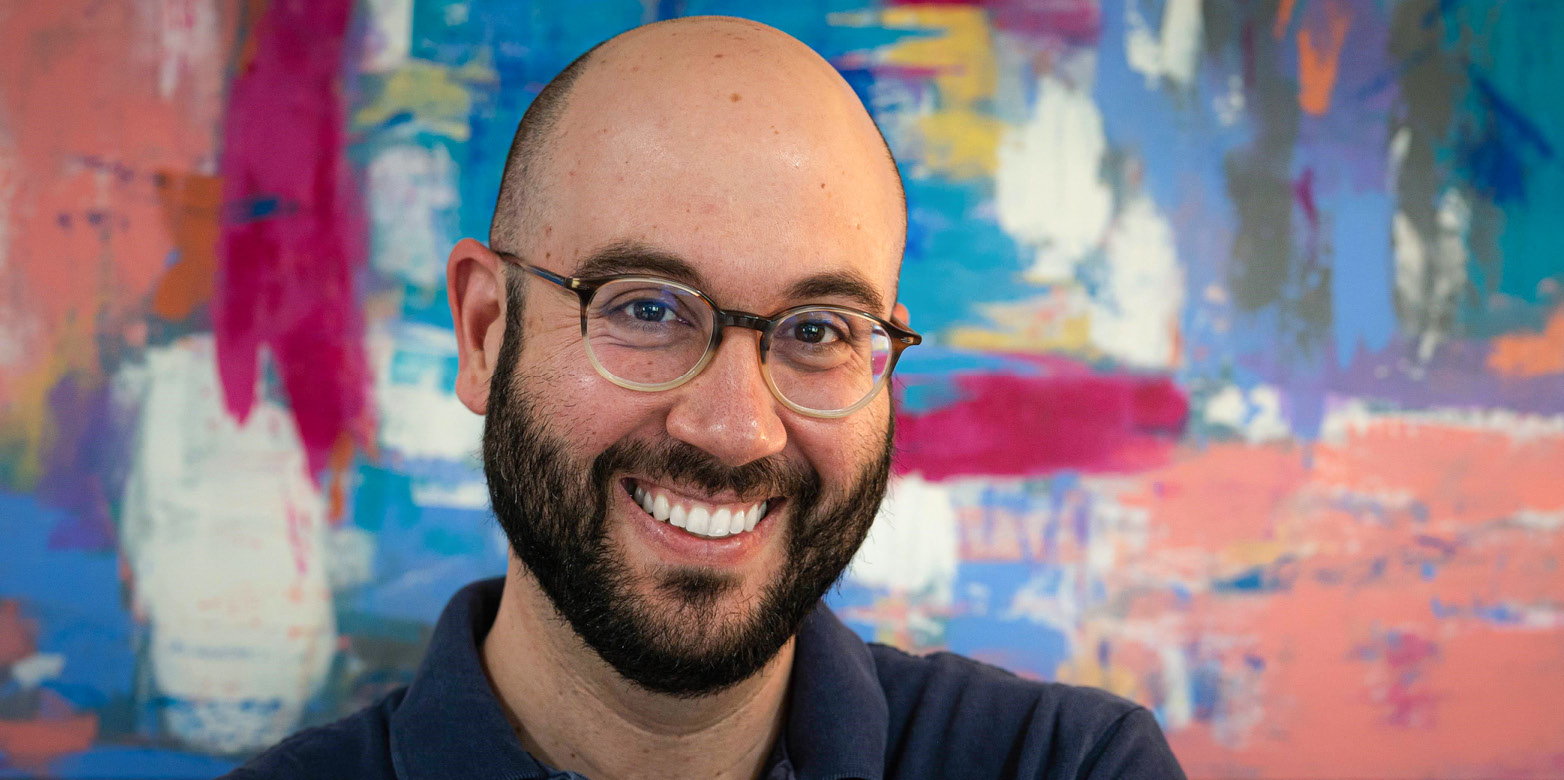
Professor Rekatsinas, welcome to ETH Zurich. What are your current research interests?
My group, the Structured Intelligence Systems Group, conducts research on algorithms and systems for intelligible, robust and scalable machine learning over complex relational data. Our long-term goal is to understand the fundamental connections between data management and modern machine learning (ML) systems. On the ML side, we work on techniques to make modern decision-making more transparent and robust to the variability and noise of data. At the same time, we develop new systems to make the use of deep learning models over billion-scale structured data faster and cheaper by attacking key bottlenecks in the ML lifecycle, such as streamlining data preparation, enabling robust and transparent development and deployment of ML models, and developing training and inference systems for resource-friendly ML.
What is the impact of your research on society?
The evolution of artificial intelligence (AI) holds a wealth of opportunities for society. From virtual assistants that provide us with constant access to information to AI models that help expedite medical diagnosis, AI holds great promise for enhancing human capabilities and making life more efficient. To this end, our research helps groups of scientists and industry partners integrate AI into different aspects of modern life more easily and reliably.
Where were you working before you came to ETH?
I started my professional career as an Assistant Professor at the University of Wisconsin–Madison where I was a member of the Database group. However, in the last few years I have been on academic leave at Apple as a lead in the AI/ML Apple Knowledge Platform team. At Apple, I helped develop solutions that power features including Siri and Spotlight. Now, I am looking forward to continuing my academic career at ETH while maintaining my strong connections with the tech industry.
Which courses will you be teaching at ETH?
As part of the Systems Group, I will be teaching various courses in the field of data management and machine learning systems. In my previous position, I designed new courses on data management for data science and machine learning that I plan to teach at ETH Zurich. The motivation behind these courses is to modernise data management education and make it immediately relevant to the fields of data science and machine learning. Introducing data management through the lens of modern analytics provides a unique opportunity to emphasise the concept of data models and to draw connections to different data processing methods along data analytics pipelines.
Name an interesting fact about your research.
My research has introduced technology that currently helps millions of people get access to higher-quality information. The HoloClean project, which I started in 2016 as a postdoctoral researcher at Stanford, was one of the first efforts to study the foundational connections between modern machine learning and data cleaning, a notorious challenge in business analytics and data integration platforms. Since then, the algorithms and ideas behind HoloClean have been commercialised and integrated into multiple production pipelines.
What do people often get wrong about your field?
Many people believe that the latest advances in AI will eliminate the need for data management and carefully designed systems. I argue that it is the exact opposite. We are actually witnessing that the model-first paradigm of ML is switching towards a data-first and systems-first paradigm. The recent breakthroughs in large-scale AI models (GPT-3, PaLM, Gato, Metaformer, etc.) have shown that scalable data processing is critical to obtaining state-of-the-art performance for tasks such as information search, image processing and text understanding. Scale comes in two forms: 1) data reconstruction workloads (such as predicting the next token in a token sequence) over vast volumes of carefully selected data and 2) efficient processing of complex computation graphs of linear algebra operations over large-scale tensors. Surprisingly, we are seeing that a large percentage of the progress in the field of AI originates from results in the field of scalable data management and processing for these new workloads.